Deep learning in High Energy Physics - Amir Farbin (UTexas at Arlington)
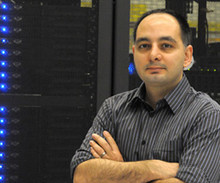
The recent Deep Learning (DL) renaissance has yielded impressive feats in industry and science, replacing laborious feature engineering with automatic feature learning, providing better algorithms, and enabling analysis of unlabeled data. DL is applicable to a large number of High Energy Physics (HEP) problems such as tracking, calorimetry, particle identification, simulation, monitoring, anomaly detection, noise reduction, data compression, workflow optimization, and data analysis. I will discuss how DL can be applied to many of these areas and overview the first attempts of DL in HEP. I will also present several public datasets that we have been compiling to enable collaborations with the Machine Learning community and contributions from the public.
La conférence est pour tout public et le café est servi dès 11h30.
Emplacement : 2900, chemin de la Tour G-715 Montréal H3T 1J6 QC Canada